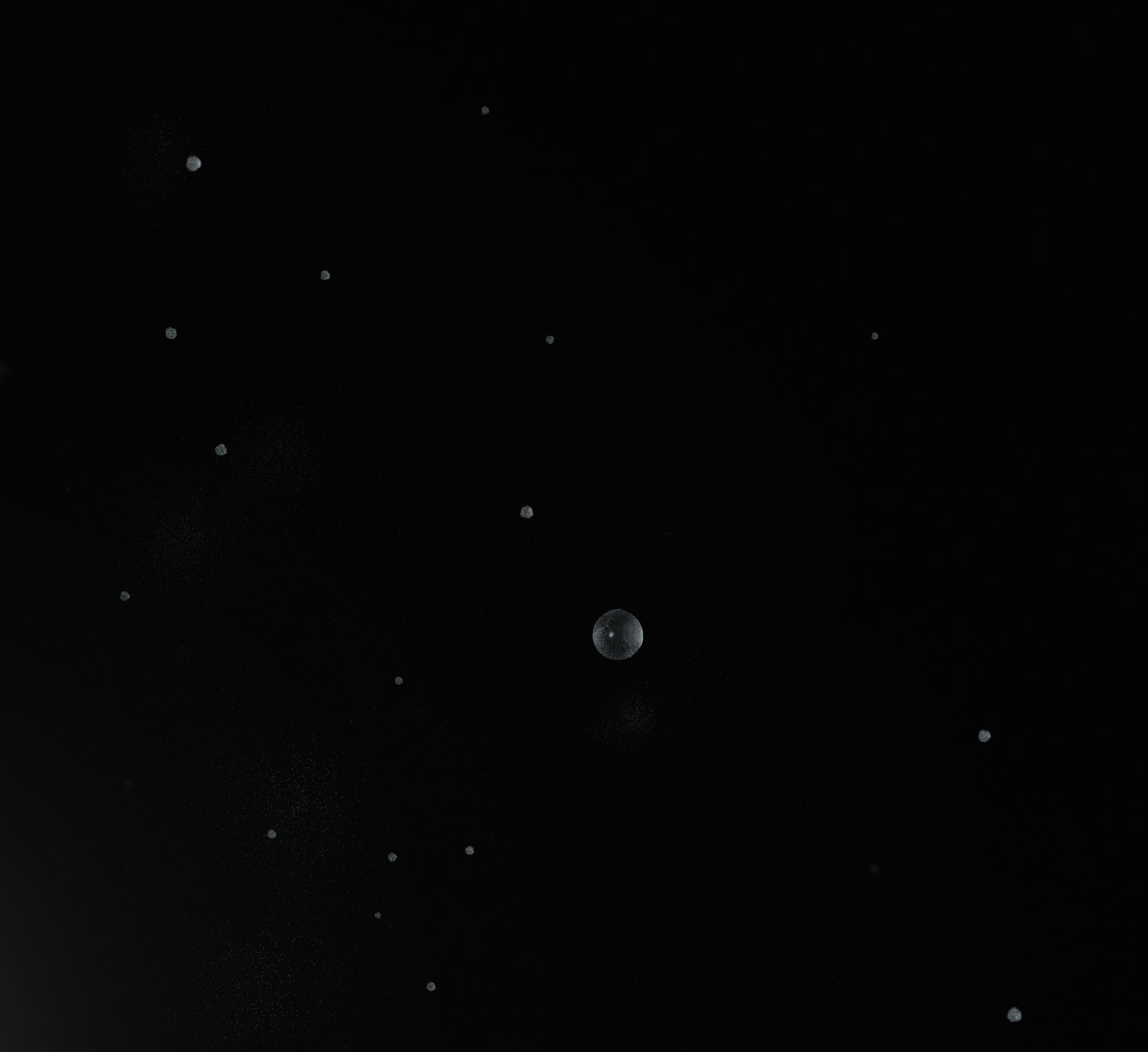
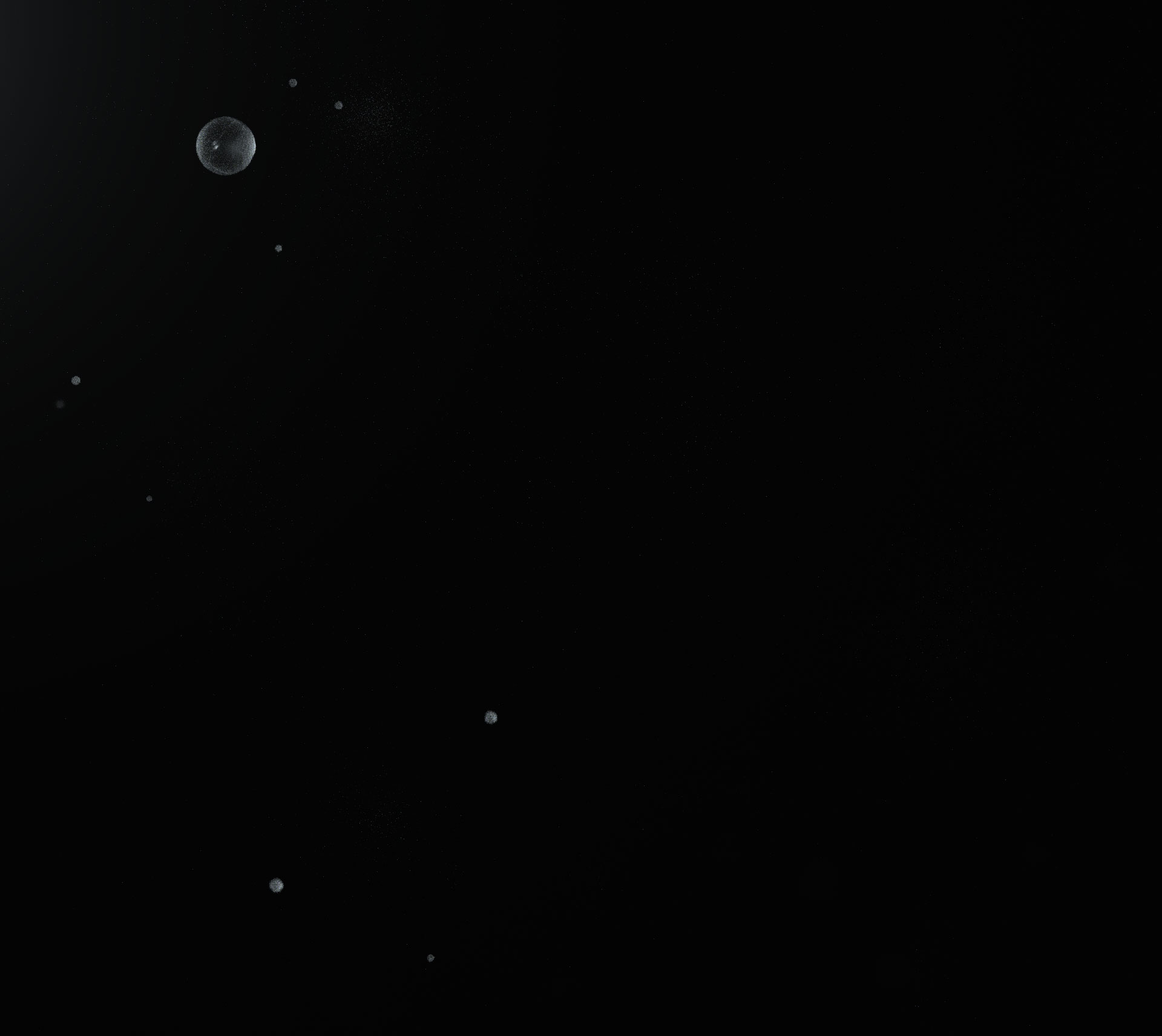

AI + CryoEM: CryoNet goes live, AI dramatically accelerates cryo-EM atomic-level model building
date:02/23/2022
AI + CryoEM: CryoNet is online, AI dramatically accelerates cryo-electron microscopy atomic model building
The revolutionary development of single-particle cryo-electron microscopy technology has enabled structural biologists to more efficiently resolve the high-resolution structures of large numbers of complex biological macromolecular machines. Building an atomic structure model from the electron cloud density map data generated by cryo-electron microscopy is the final step in cryo-electron microscopy structure, and currently relies heavily on experienced structural biologists using manual plus software-assisted approaches. This is a demanding task, requiring the model builder to have a high level of knowledge of protein structural features and side chain conformations, and mastering these knowledge and laws requires a lot of time to learn and practice. Especially for regions with low local resolution, manual construction may take a lot of time for refinement, and even additional experiments are needed to obtain higher resolution density maps for resolution. Rapid, accurate, and automated construction of atomic models from electron cloud density maps is a key step in liberating cryoelectron microscopy productivity.
Home page of CryoNet
CryoNet is an artificial intelligence algorithm combining 3D computer vision and natural language processing, based on the attention model for amino acid identification from frozen electron density maps and building atomic models (Figure 1).CryoNet is trained with a large number of resolved atomic structure models and matched electron cloud density maps: learning the internal atomic structure of amino acids and The atomic models are accurately identified from the frozen electron cloud density map by learning the correspondence between amino acid sequences and electron cloud density features through the attention model; the correct generation of side chain conformations is achieved by adding a forward kinematic model with bond length and bond angle constraints to the algorithm. In addition, CryoNet can iteratively perform structural fragment complementation and fine-tuning, further enhancing the accuracy of the atomic models. examples of CryoNet prediction results are shown in Figure 2.
Method of CryoNet
Example of CryoNet predicted results
CryoNet website: https://cryonet.ai
Users can submit electron cloud density maps and amino acid sequences directly without registration, and CryoNet will build the all-atom model and provide it for download.
Alternatively, CryoNet can also build all-atom models directly from the electron cloud density map when amino acid sequences are not available.