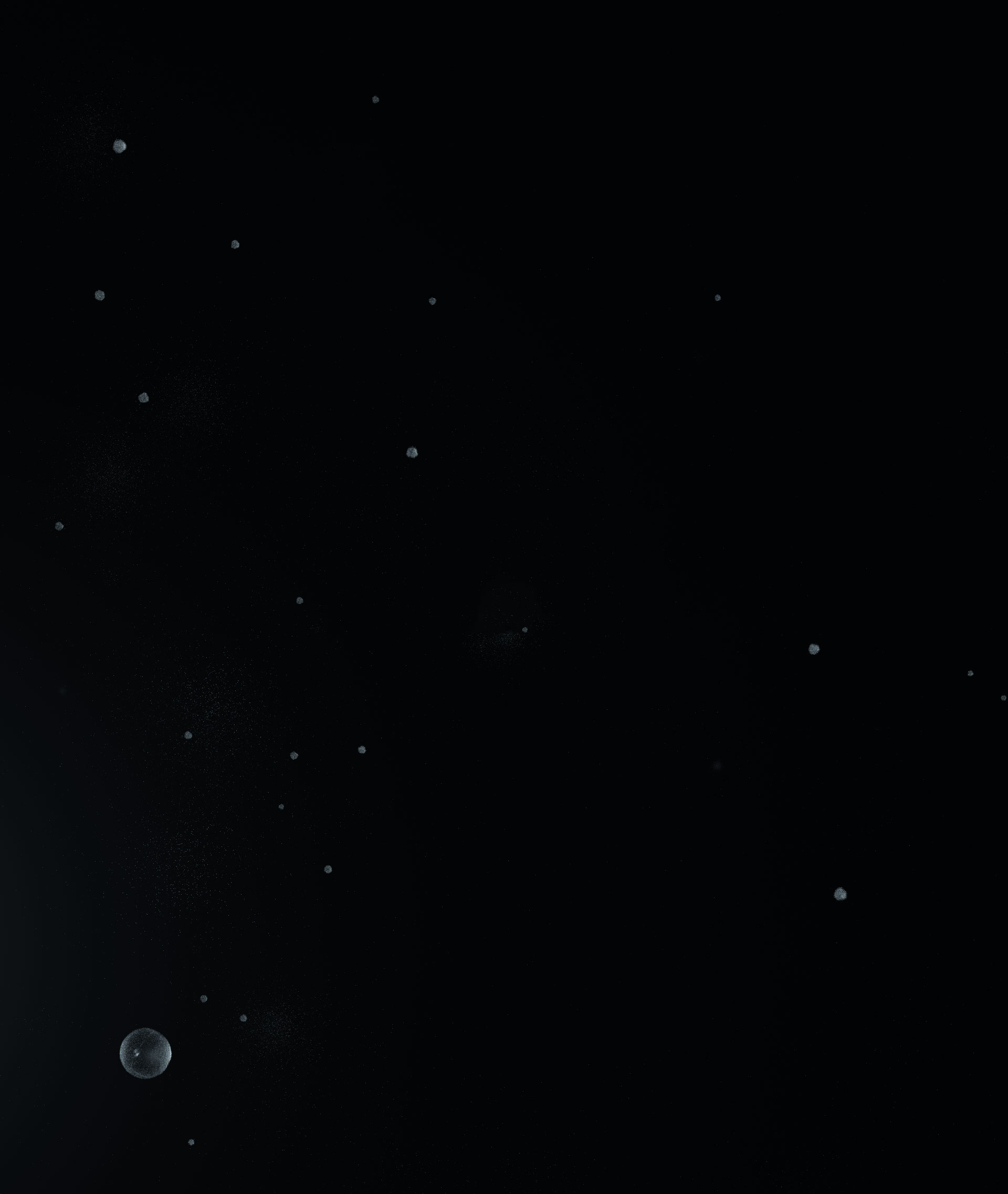
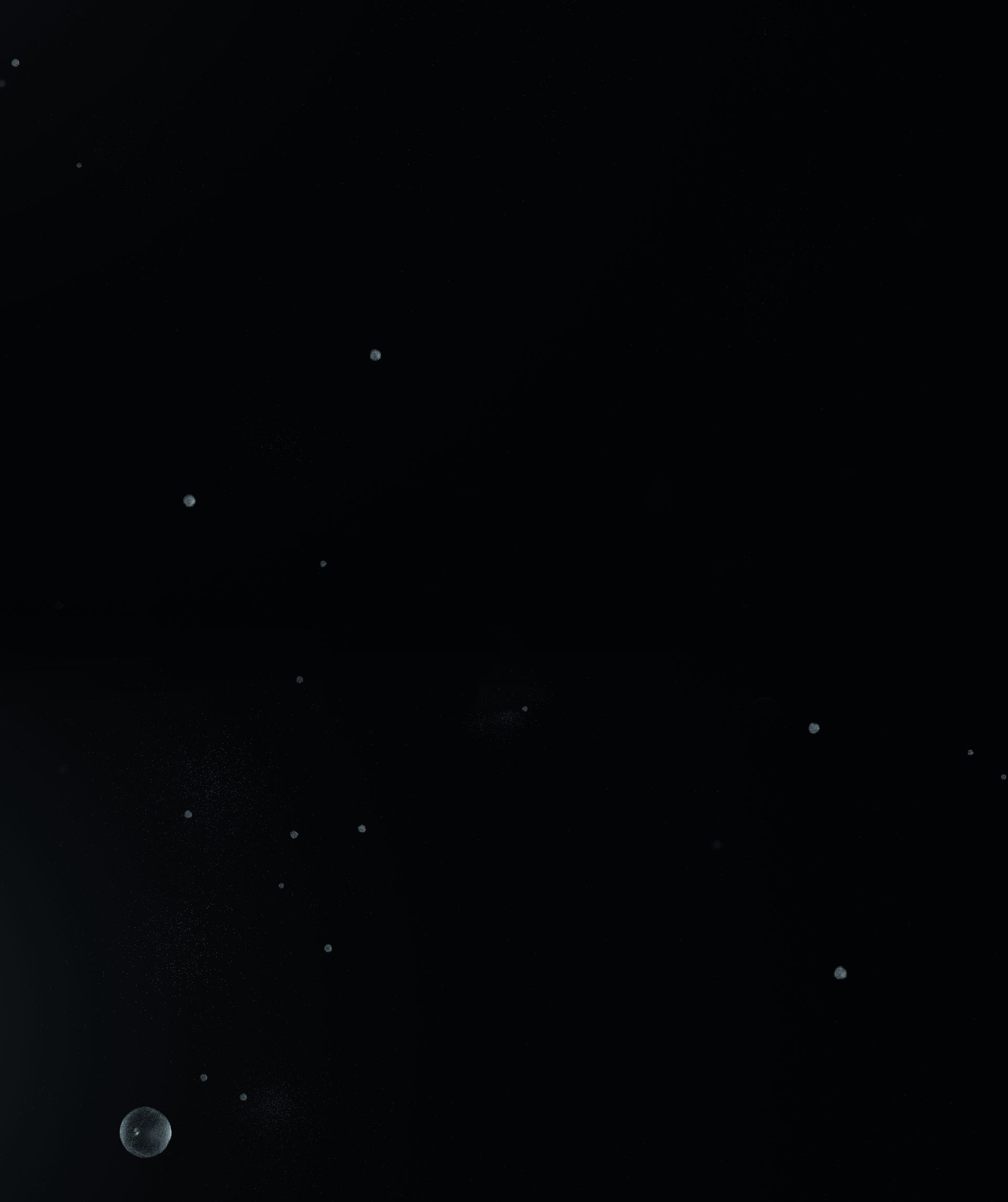
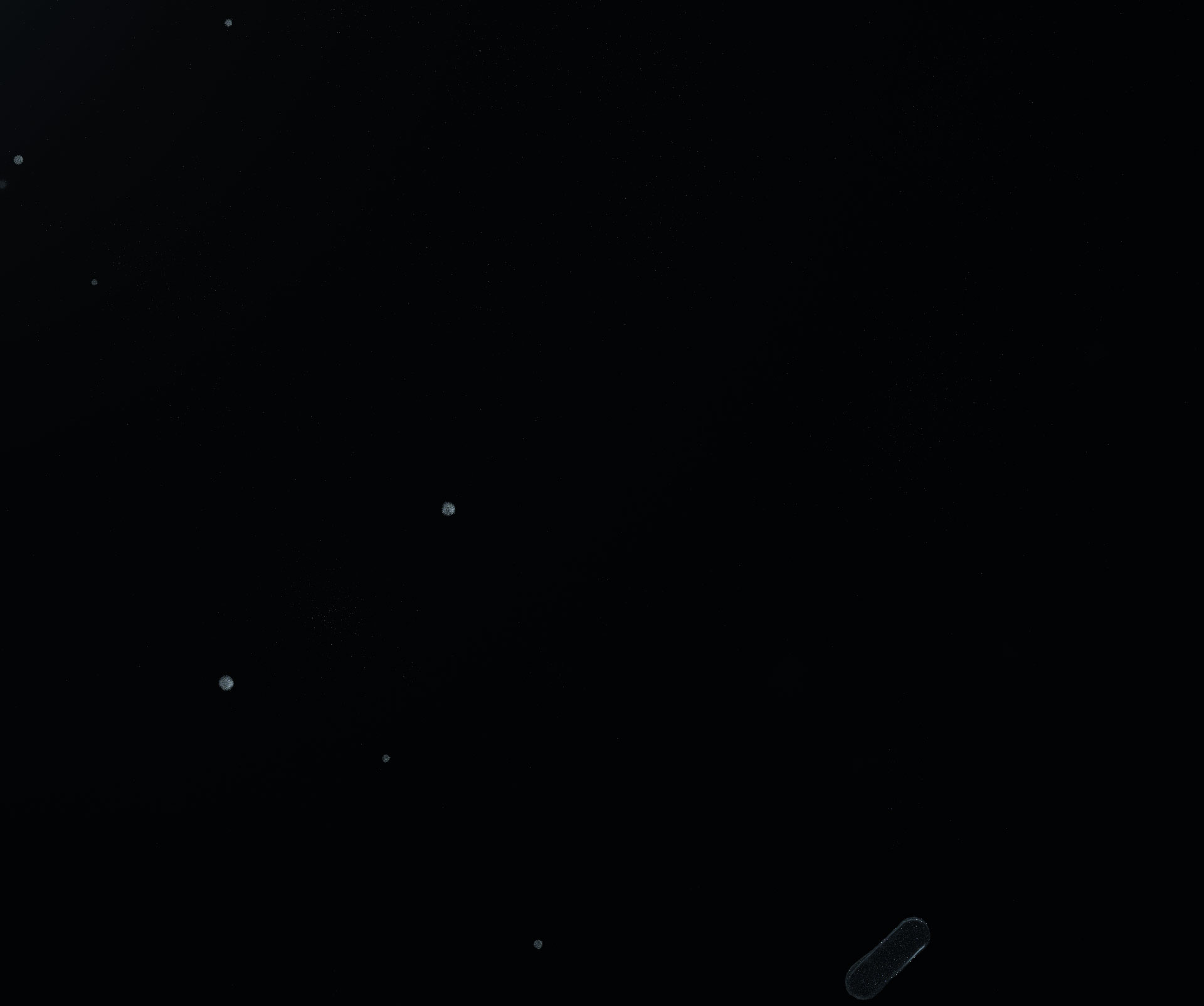
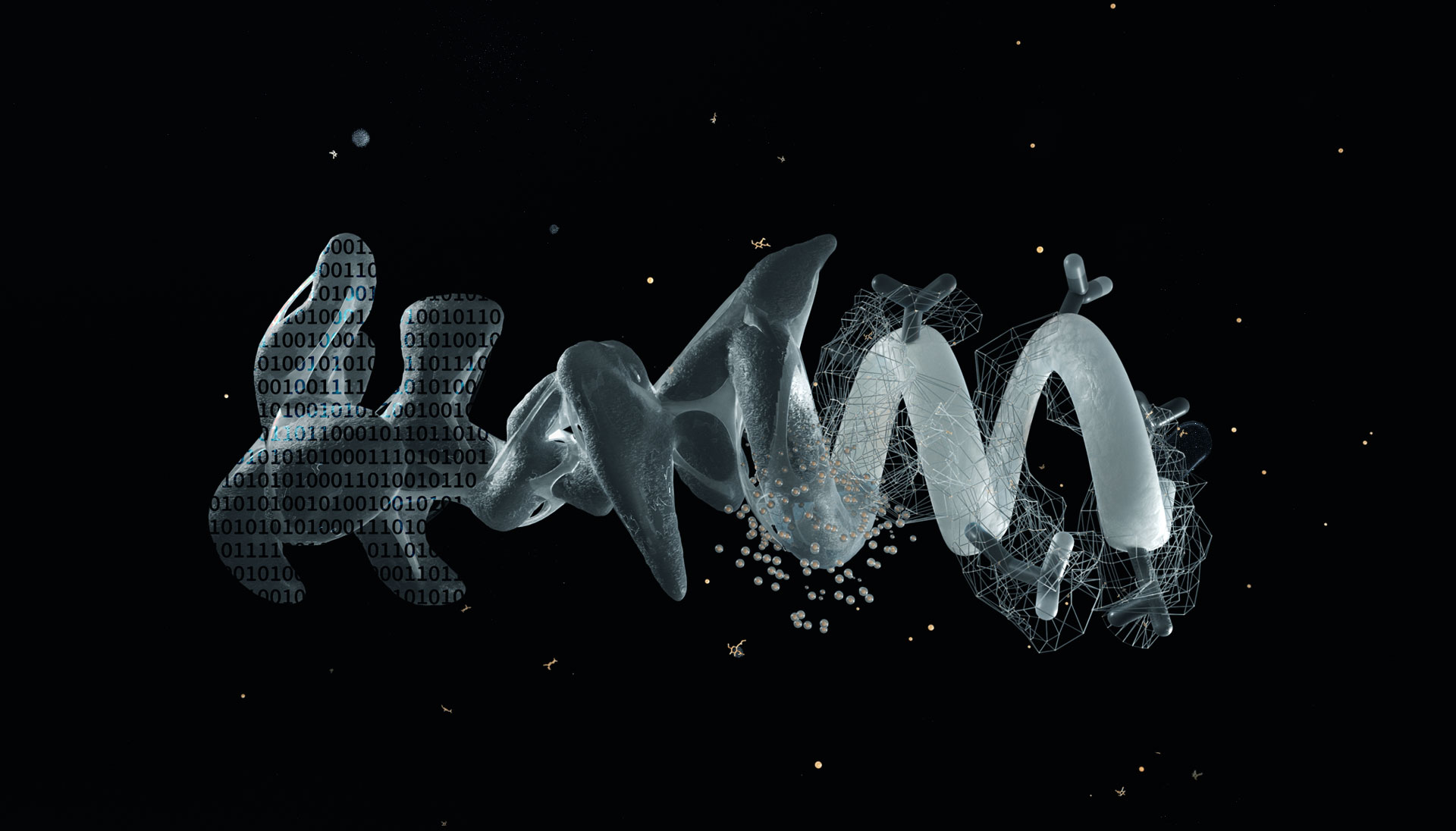
TECHNOLOGY - CryoNet
B A C KCryoNet Cryo-EM Modeling
with Deep Neural Network
A new fully differentiable neural network based method to directly identify the 3D atomic model from cryo-EM density map in only few minutes without any human intervention.
Molecular model building as the critical last step remains a bottleneck, despite the progresses towards automatic.
Manual operation is time-consuming and inevitably error-prone. Significant incorporation of automation has resulted in the ability to obtain near-atomic resolution reconstructions within hours to days. However, molecular model building is difficult to automate since it relies substantially on human expertise, which limits scalability for use by wider community. A model that represents the atomic coordinates of each amino acid is constructed and fitted into the 3D electron density volume as the final step. Specific amino acids to a density volume are manually assigned by structural biology experts with the help of 3D visualization tools. Attempts to automate this process are far from satisfactory given to the accuracy, coverage, time-consuming and requirement of human intervention.
As part of the push to improve efficacy and performance of model building, Pro Qiangfeng Zhang (one of ShuimuBio scientific advisor) has proposed a learning-based method from a totally novel perspective. The power of deep convolutional neural net-work is leveraged to develop a novel framework for amino acid detection. And a sequence-guided Monte Carlo Tree Search (MCTS) is designed to thread over the candidate amino acids to form the molecular structure.
AI-driven: hundreds of times faster and several times more accurate than existing automated solutions without any human intervention.
These endeavor for better model building is expected to fuel the rapid growth of cryo-EM atomistic structures. We hope our exploration will facilitate the integration of machine learning and more collaboration with experts from fields outside of traditional structural biology.
● This is the first attempt to formulate molecular structure determination from Cryo-EM density volumes with a deep learning approach.
● We adapt a novel 3D network architecture for amino acid detection and internal atom coordinate estimation in density volumes, and proposed an APRoI layer and neighbor loss for better performance.
● We design a sequence-guided MCTS algorithm for fast and accurate main chain threading.
● We will release a large scale, richly annotated dataset of protein density volumes, to facilitate research in this area.